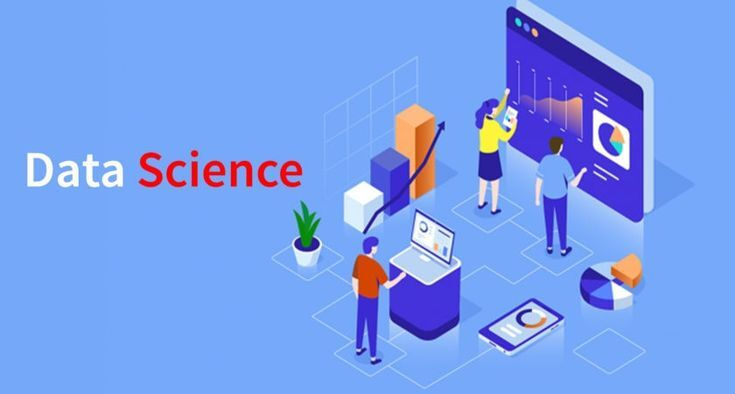
Data is central to analytics, driving decisions, improving processes, and generating value. However, not all data is the same. Different data types require unique techniques and tools for analysis. Understanding these data types is crucial for anyone working in data analytics, ensuring the data is processed and interpreted accurately.
This article explores the fundamental data types used in analytics and highlights their importance in data analysis.
What Are Data Types?
Data types classify data based on the kind of value a variable holds and the operations that can be performed on it. Using the right data type is critical to applying appropriate analytical methods and algorithms. Misinterpreting data types can lead to incorrect conclusions and inaccurate results.
Why Are Data Types Important in Analytics?
Choosing the correct data type ensures:
Accurate data collection
Effective analysis methods
Reliable results
Efficient storage and processing
Data types also affect how data is visualized and communicated. Different data types are best suited for specific chart types, graphs, and tables.
Major Data Types in Analytics
Analytics primarily deals with four main categories of data types: numeric, categorical, ordinal, and date/time data. Each plays a distinct role in analytical processes.
1. Numeric Data
Numeric data consists of numbers that can undergo mathematical operations. This type is divided into two subcategories:
a. Integer Data
Integers are whole numbers, which can be positive, negative, or zero. For example, the number of products sold, customer age, or website visits are integer data.
b. Floating-Point Data
Floating-point numbers, or decimals, represent numbers with fractional components. They are useful when more precision is needed, such as in prices, scientific measurements, or percentages.
Importance of Numeric Data in Analytics
Numeric data forms the backbone of many analytical models, including predictive analytics, machine learning, and statistical methods. It is used to calculate averages, identify trends, and find correlations, helping businesses make data-driven decisions. Proper handling of numeric data is crucial for accurate analysis, especially in finance, where small errors can lead to significant consequences.
2. Categorical Data
Categorical data consists of labels or categories that cannot undergo mathematical operations. Also known as qualitative data, it is often classified into nominal and ordinal types.
a. Nominal Data
Nominal data comprises categories without inherent order, such as gender (male, female), product types (electronics, clothing), or regions (North, South, East, West).
b. Ordinal Data
Ordinal data is similar to nominal data but includes a clear order or ranking system. Examples include customer satisfaction levels (poor, fair, good, excellent) or education levels (high school, bachelor's, master's).
Importance of Categorical Data in Analytics
Categorical data is essential for segmenting and grouping information. For instance, customer data categorized by age or region can reveal trends specific to particular demographics. This type of data is crucial for customer analysis, market segmentation, and identifying trends.
3. Ordinal Data
Although ordinal data is a subtype of categorical data, its ranking system makes it worth addressing separately. It is commonly used in situations where the relative order of categories matters, such as rating scales or educational levels.
Importance of Ordinal Data in Analytics
Ordinal data is often used in surveys and questionnaires, where the order of responses provides valuable insights. For example, understanding how customers rank their satisfaction with a product helps businesses improve their services.
4. Date/Time Data
Date and time data represent specific dates, times, or durations. This data type is essential for time-series analysis, where trends are examined over time. Examples include the date of purchase, time of an event, or call duration.
Importance of Date/Time Data in Analytics
Time is a critical factor in many analyses, such as trend analysis, seasonality, forecasting, and historical comparison. Proper management of date and time data allows organizations to monitor performance over time and make informed predictions.
Other Key Data Types in Analytics
Apart from the primary types mentioned above, some fields require specialized data types that need specific handling:
1. Boolean Data
Boolean data represents one of two possible values: True/False or Yes/No. It is commonly used when a binary decision is required, such as whether a customer completed a purchase or if a product passed a quality check.
2. Text Data
Text data, also known as string data, consists of characters or words and is often found in unstructured or semi-structured formats like social media posts, product reviews, and customer feedback. Natural Language Processing (NLP) techniques are often employed to extract insights from large volumes of text data.
Data Types and Data Science
Understanding and classifying data types accurately is essential in any data science project. Misinterpreting data can lead to flawed models and insights, negatively affecting business decisions. For instance, treating categorical data as numeric may result in misleading trends and analyses, leading to incorrect predictions.
Learning how to handle different data types is crucial for data science training, particularly at a Data Science Training Institute in Patna and all cities in India. A strong grasp of numeric, categorical, and date/time data is foundational for effective analysis.
Conclusion
In analytics, data types form the basis of data processing and interpretation. Understanding these types—numeric, categorical, ordinal, date/time, boolean, and text—ensures that the correct analytical methods are applied, leading to accurate and actionable insights. Properly managing and interpreting data types is essential for any successful data analysis project, regardless of the industry.
For students and professionals attending a Data Science Training Institute in Patna and all cities in India, learning how different data types influence analysis is crucial for building strong foundations in data science and analytics.
Comments